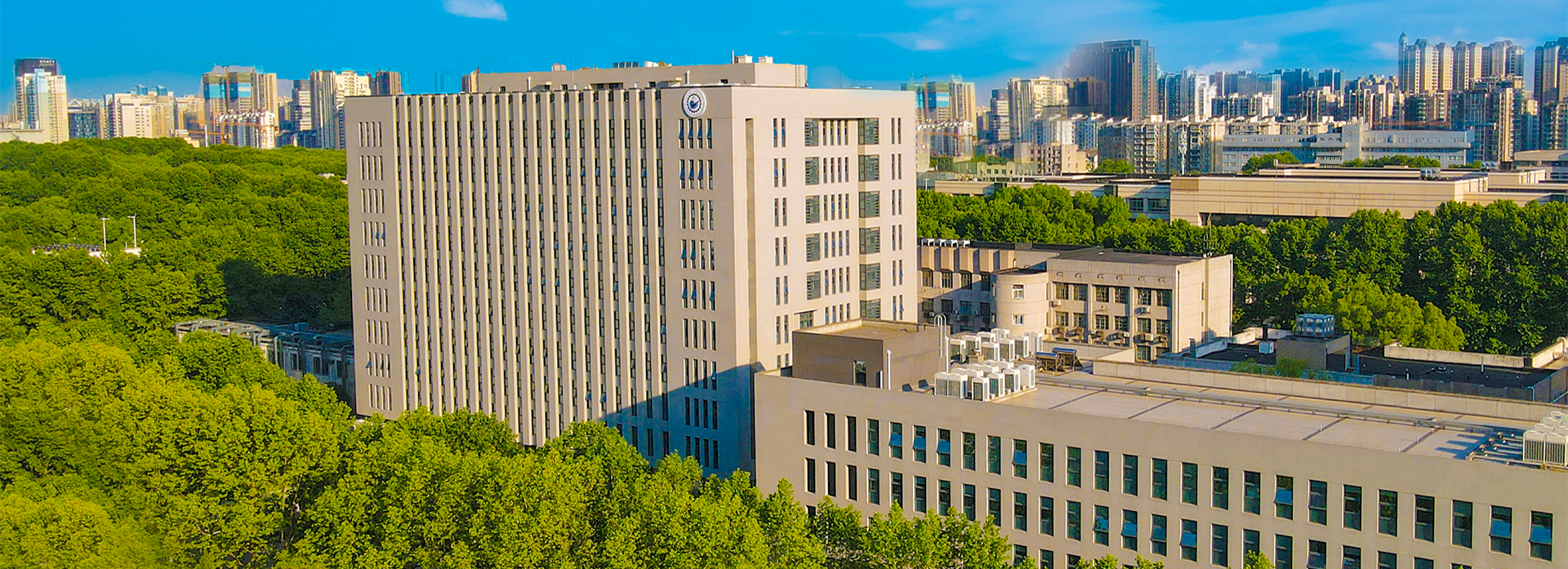
Zimin Yang, Xiaosheng Peng, Jiajiong Song, et al. Short-Term Wind Power Prediction Based on Multi-Parameters Similarity Wind Process Matching and Weighed-Voting-Based Deep Learning Model Selection[J]. IEEE Transactions on Power Systems, doi: 10.1109/TPWRS.2023.3257368.
Yuzhu Chen, Xiaosheng Peng , Hongyu Wang, et al.Generator Stator Partial Discharge Pattern Recognition Based on PRPD-Grabcut and DSC-GoogLeNet Deep Learning[J]. IEEE Transactions on Dielectrics and Electrical Insulation, doi: 10.1109/TDEI.2023.3275548.
Xiaosheng Peng, Zimin Yang, Yinhuan Li, et al. Short-Term Wind Power Prediction Based on Stacked Denoisedauto-Encoder Deep Learning and Multi-Level Transfer Learning[J]. Wind Energy, doi: 10.1002/we.2856.
Xiaosheng Peng, Cong Li, ShiyuanJia, et al. A short-term wind power prediction method based on deep learning and multistage ensemble algorithm[J]. Wind Energy, 2022, 25(9): 1610-1625.
Ruiqin Duan, Xiaosheng Peng, Cong Li, et al. A hybrid three-staged, short-term wind-power prediction method based on SDAE-SVR deep learning and BA optimization[J]. IEEE Access, 2022, 10: 123595-123604.
Xiaosheng Peng, Yinhuan Li, Lie Dong, et al. Short-Term Wind Power Prediction Based on Wavelet Feature Arrangement and Convolutional Neural Networks Deep Learning[J].IEEE Transactions on Industry Applications, 2021, 57(6): 6375-6384.
Xiaosheng Peng, Hongyu Wang, Jianxun Lang, et al. EALSTM-QR: Interval wind-power prediction model based on numerical weather prediction and deep learning[J].Energy, 2021, 220.
Xiaosheng Peng, Qiyou Xu, Hongyu Wang, et al. A novel efficient DLUBE model constructed by error interval coefficients for clustered wind power prediction[J].IEEE Access, 2021, 9: 61739-61751.
Zhendong Zhang, Chao Wang, Xiaosheng Peng, et al. Solar radiation intensity probabilistic forecasting based on K-Means time series clustering and gaussian process regression[J]. IEEE Access, 2021, 9: 89079-89092.
Jianxun Lang, Xiaosheng Peng, Wenze Li, et al. A novel two‐stage interval prediction method based on minimal gated memory network for clustered wind power forecasting[J]. Wind Energy, 2020, 24(5) : 450-464.
Xiaosheng Peng, Yuzhu Chen, Kai Cheng, et al. Wind power prediction for wind farm clusters based on the multifeature similarity matching method[J]. IEEE Transactions on Industry Applications, 2020, 56(5): 4679-4688.
Xiaosheng Peng, Fan Yang, Ganjun Wang, et al. A convolutional neural network-based deep learning methodology for recognition of partial discharge patterns from high-voltage cables[J].IEEE Transactions on Power Delivery, 2019, 34(4): 1460-1469.
Xiaosheng Peng, Jinshu Li, Ganjun Wang, et al. Random forest based optimal feature selection for partial discharge pattern recognition in HV cables[J]. IEEE Transactions on Power Delivery, 2019, 34(4): 1715-1724.
Xiao Hu, W. H. Siew, Martin D. Judd, Xiaosheng Peng. Transfer function characterization for HFCTs used in partial discharge detection[J]. IEEE Transactions on Dielectrics and Electrical Insulation, 2017, 24(2): 1088-1096.
Xiaosheng Peng, Jinyu Wen, Zhaohui Li, et al. SDMF based interference rejection and PD interpretation for simulated defects in HV cable diagnostics[J]. IEEE Transactions on Dielectrics and Electrical Insulation, 2017, 24(1): 83-91.
Xiaosheng Peng, Jinyu Wen, Zhaohui Li, et al. Rough set theory applied to pattern rrecognition of partial discharge in noise affected cable data[J].IEEE Transactions on Dielectrics and Electrical Insulation, 2017, 24(1): 147-156.
Xiaosheng Peng, Chengke Zhou, Donald M. Hepburn, et al. Application of K-Means method to pattern recognition in On-line cable partial discharge monitoring[J]. IEEE Transactions on Dielectrics and Electrical Insulation, 2013, 20(3): 754-761.